Qualitative research
Schottler Consulting Pty Ltd
Social and market research services

Australia / NZ company headquarters
L38, 71 Eagle St Brisbane 4000
+61 7 3166 9096 Mon-Fri 8am-6pm
Schottler Consulting Social and Market Research Knowledge Centre
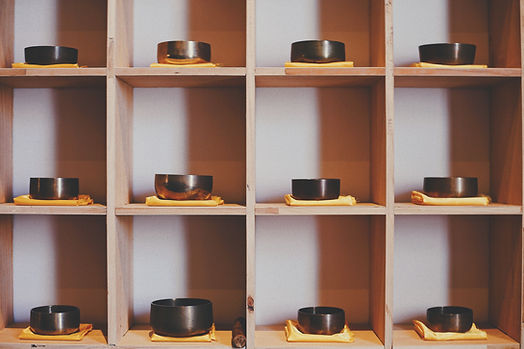
How should I be storing and labelling my social or market survey data?
​
In conducting research for well over a decade, our company has witnessed a range of very poor data storage procedures across organisations involved in market or social research.
​
Probably the most significant problem has been the difficulties associated with poor labelling of data sets. On many occasions, we have opened-up data sets from past research only to find very confusing variable labels or data labels poorly described.
This can make use of past data very difficult, given the challenge of identifying which variables correspond to which survey measures (especially in complex data sets!).
Surprisingly, in many cases, we have found that some survey variables have no labels at all, implying that researchers have to largely take a “best
guess” at which variable is which.
This also adds to much frustration and extra time required to untangle data to work out which questions in the survey correspond to which variables in the data set.
​
Tips for success in storing and labelling social and market research data
​
For this reason, we recommend and follow a series of best practice steps for data storage where possible and practical.
​
1. Match variables labels to survey measure labels – while a seemingly simple principle to follow, numbering survey questions and using the same numbers in the data set (variable labelling) is the best way to avoid confusion over 'which variable is which' down the track.
In practical terms, survey question 1A and1B, should ideally also be labelled with the same 1A or 1B numbering in the variable labels.
This avoids so many issues, but despite its simplicity, few organisations seem to follow this straightforward principle.
​
​
2. Add a description to the data variable which exactly matches the survey question – if the survey variable measures 'frequency of visiting the doctor each week', then logically use this same exact label – particular attention should be placed on capturing all necessary information (e.g., not just visiting the doctor, but visits per week) to allow anyone to understand the variable in the data set without having the survey on hand
​
3. If data is recoded or new variables are added to the data set (e.g., perhaps a new variable which sums the total of several other variables), ensure that the new or recoded variables are correctly labelled with information on HOW the variable recode or transformation was performed.
In this respect, it can be quite difficult to understand how a recoded variable was derived without such information.
In every case, it is also good practice to keep the recode syntax to allow researchers to look at specific recode commands.
​
It is good to store your data as a master set including:
1. The survey instrument
2. The raw data set with all labelling as described above
3. A summary of variables + codes (these can often be automatically be generated from packages such as SPSS statistical package)
​
4. Syntax information on variable recodes and new variables
5. Qualitative open-end responses should ideally also be included in the same data set containing the raw data – this also allows easier linking of qualitative and quantitative data in the data set.